What is AIOps? How AIOps Technology Can Benefit IT Operations? Explained
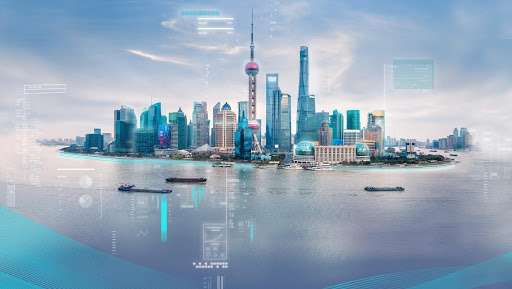
What is AIOps?
AIOps stands for stands for “artificial intelligence for IT operations.” It means incorporation of analytics and machine learning for balancing and enhancing different types of IT operations. AIOps is perceived as continuous integration and development (CI/CD) for basic IT operations. In addition, it boots two prime components which include big data and machine learning (ML).
The
Originally coined by Gartner researchers, the term AIOps was initially termed as “Algorithmic IT Operations”. However, the term was later renamed to “Artificial Intelligence for IT Operations” seeing its increase in adoption and popularity of AI and deep interest of IT firms in the technology. It is defined as the way through which data and information are being used by the IT team from an IT environment by using AI and MI. As per the Gartner report on AIOps, it is predicted that by 2022, almost 40% of the IT firms will start making use of AIOps to monitor, automate and perform other different tasks.
In fact, some of the organizations have already adopted technology and offering efficient services to their customers. Some of the leading AIOps platforms include – Elastic, Splunk ITSI, Opsgenie, BigPanda, and SumoLogic.
The prime role of AIOps is to redesign conventional IT processes and tool that are not in sync with the industry’s current demands. Businesses require a transit from tools, lack of interoperability, and siloed databases for cost-effective operations and manage reoccurring changes in the budgets.
The amazing features and capabilities of AIOps have positioned it as the future technology infrastructure that would be embraced by many companies ranging from small-sized to large-sized. As businesses are in need to shift from the conventional methods of IT operations. They are also in growing need to have a specific tool to timely identity problems through accurate assessment of the behavior of infrastructure. The AIOps platforms are designed to monitor behaviors of the edge technology and establish cost controls by dynamic analysis of public cloud utilization.
Machine Learning and Human Limits within the Organization
The human mind is trained to take short-cuts and artificial intelligence is designed to take comprehensive routes for solving any problem. This is the main difference that distinguishes human brains and machine learning. It is also one of the reasons behind the capabilities of machine learning to overcome human limitations.
Whenever we are exposed to a situation where we are required to make decisions that are more like life and death calls we follow our heart or guts as we normally say it.
But machine learning or AI in particular neither has a heart of guts as it has a computing device fit within it that does all the calculation to figure out the end result.

Machine learning evaluates extensive data in a fraction of minutes or even seconds and draws a conclusion from it. The human mind is capable of doing the same but it would take it days, months or even years depending on the volume of data to analyze and draw a conclusion.
Besides, while going through the data, the human mind may miss out certain crucial information which AI or AIOps infrastructure will never do. Here comes the role of AIOps in overcoming the limitations of humans.
Machine learning has turned out to be effective in spotting weak signals, shifting from text to visualizations for better comprehension of the complexities. Using the machine learning tools we can diagnose illness, prescribe a treatment, avoid conflict, plan capital, invest money, and appoint an advocate and do a lot more.
The Core Elements of AIOps Platforms and Its Use Cases
The necessary components that make up an AIOps platform include –
- Machine learning – It can help in automatically structuring and creating new algorithms by analyzing the newly introduced data and considering the output of algorithmic analysis into the system
- Performance baselining – It includes usage of AI to pin all the baseline metrics that can affect the performance of the application,
its infrastructure and services offered. All the factors affecting the system are checked thoroughly from the back and front end. - Anomaly detection – This will spice up the monitoring with AI. The detection of abnormal metric values can help in identifying undetected issues in the stack. The detection of the issue in advance can reduce the perimeter of the search space that is further required to diagnose a problem.
- Automated root cause analysis – Under this, the outcome generated by the AI or machine learning will be utilized to automatically create and add a response or update the issues and other situations that are identified on the platform.
- Predictive insights – It involves the extraction of data from existing data sets so as to identify future trends and patterns. It utilizes machine learning, artificial intelligence that can help IT firms to deliver proactive support services.
Once the above-mentioned components are applied correctly within the organization, AIOps can be used in the following use cases –
- Detection of the anomaly – Using advanced machine learning algorithms; it becomes easier for IT teams within the organization to automatically trigger alerts at the time when anomalies indicate a potential issue by detecting different patterns in data. For example, Logstash is being delivered Elastic’s ELK X-pack with built-in machine learning.
- Alarm management – Operations teams within the organization make use of certain alert and monitoring tools to keep a check of the services offered. These tools many a time led to a flood of alerts which can be annoying and filled with noise. Thus, to overcome such behavior, AIOps can be used as an automatic responder to correlate and provide alert to the situation much before human responders become active. BigPanda L0 is a perfect example of such an application.
- Causal Analysis and Visualization – SRE and DevOps team within the organization are usually dependent on real-time metrics collected from the datasets to get familiar with the value of the services offered. Causal analysis can help in building more complex metrics to find out their dependence on services and systems. Comparing both, the DevOps personnel are given a rough visualization that can help them to stay ahead of their competitors. A good example of this is a Splunk ITSI’s glass tables.
- Intelligent remediation – Intelligent remediation is a process that depends upon data sets, machine learning, and artificial intelligence to work on issues faced by the DevOps team. Intelligent remediation is different from manual remediation under which one has to wait for human engineers to perform actions in responding to an incident. However, in the case of intelligent remediation, since all the actions are automated using MI and AI, issues faced by the DevOps team are solved more quickly to give users a better experience.
How AIOps Technology Can Benefit IT Operations?
Adoptions of AIOps can let IT organizations to enjoy benefits like –
- Managing storage efficiently – Since most of the data will be stored in the cloud, MI and AI will ensure that data sets are properly organized and secured, to be used later by higher authorities. Apart from that, through predictive analytics, storage capacity is also adjusted between the servers as new volumes are added proactively.
- Better decision making – Features of AI and MI within the AIOps platforms have the potential to effectively boost the decision-making capacity within the enterprise. The insights obtained by considering the internal and external sources through machine learning and artificial intelligence ensures that the leaders of the organizations can make better and faster decisions.
- Fully utilizing the available resources within the organization – Applications can automatically scale-in and scale-out because of the elasticity that cloud adds to the infrastructure in the firm. This scaling can be managed by keeping a check on different parameters such as the consumption of CPU, tasks standing in the queue etc. With AIOps, administrators scale out different tasks in real time because of AI that adjusts automatically keeping a check on historical data.
- Capacity planning – Despite the application of cloud infrastructure, one might think of capacity planning becoming dynamic but architects within the organization still consider it a major flaw when it comes to uploading work into the right servers and VM configurations. However, with the application of AI, everything can be mapped to the right server, thereby eliminating the chance of any errors.
- Analyzing and detecting threat – AIOps plays a vital role in enhancing the security of IT companies. By making use of heuristics and machine learning algorithms different risk events and anomalies can be detected and kept at bay. Application of AI within the organizational infrastructure can help in detecting a variety of violations and cyber attacks. Data and information obtained from within the organization can be combined with external threat intelligence feeds including domains, malicious IP’s to prevent a data breach.
- Cost Reduction – Performance issues and outages in the major issues that affect almost every business. Therefore there is a need for every business organizations to reduce the mean time to resolution (MTTR) to survive in the long-run. With the integration of AI, there is a possibility of reducing MTTR and further preventing emerging issues. All such things can help in reducing the costs since issues related to the performance problems will be eliminated.
Five Real-World Examples Using AIOps Technology
Machine
- Netflix: The best deployment of ML has been with Netflix. Using the ML tailored technology users can personalize feed and get recommendations based on their preferences. For humans, it is near
to impossible to remember the preferences of millions ofthe Netflix users. Around 80% of the entire platform operates on the recommendations made by the ML tool. The ML tool which is also the central tool of the platforms maintains a track on the user behavior as well as program content. The data thus gathers is merged to create recommendations for the users. - Siri & Cortana: The Siri and Cortana, no matter how annoying they can be for someone, are again a magnificent result of AI application. The voice recognition tools also known as deep neural networks have been trained to imitate human interactions and carry out commands. Through the interaction, the tools develop an understanding of the grammar of the language and follow up the command. Humans are for sure are not capable of doing so.
- Google: The search engine giant needs a few keywords to help you with your research. Do you think a human is capable of doing the same? Certainly not! The tool uses ML to analyze trillions of the website on the internet in a fraction of second and reach to the final result without making you wait. It expanded its horizon by introducing Gmail, Google photos, Google drive and a lot more. Many businesses are using
its services to better communicate, manage and store data, and carry out business operations effectively. The Gmail, which is also an ML-based platform for sharing emails, can be used to create personalized emails, greetings and a lot more. No man on the earth has been bestowed with these qualities. - Uber: Uber uses ML technology for tracking the location of the passenger and determining all the possible routes to reach the desired location. Uber has a special ML tool placed that helps in analyzing the movement of a particular passenger on his previous trip. Uber Eats also uses a similar platform for establishing an estate on delivery and preparation time.
- Spotify: Spotify is another ML-based tool that works just like Netflix. It tracks the playlist of the user and recommends songs and music based on the music previously played by the particular user.

Computers are more capable than ever and have reduced human limitations and bias completely. The ML and AI technology are most likely to revolutionize the conventional way of business operation without hampering much of the workforce. With swift adoption of the ML and AI it is obvious for anyone to be afraid of losing one’s job. However, the truth is ML is incomplete with humans. It is designed and trained by humans and also has certain risks of damage and breakdown which may adversely affect a business in the absence of humans. ML and AI can help enhance the perception of human enabling them to make decisive decisions based on the analysis of the huge volume of data carried out by the AI. AI should be perceived as a way to improve the effectiveness of a business, development, applications and IT infrastructure.
The Need of AIOps In Future
It is predicted that by this year, at least one in four global enterprises will begin the adoption of AIOps platforms for the majority of its IT operations. As businesses grow the IT infrastructure will witness a gigantic change in scale. Any company on the growth trajectory needs AIOps to tackle the overload of the data warehouse. The growing volume of development and operation processes it is likely to see that people will feel more tired and fatigued than ever. AIOps can come up as a great help for a business willing to stay competitive in the industry. It can also prove helpful in automating codes requires by CI/CD developers as per their CI/CD pipeline. With the automated codes, the developers can better monitor and analyze as well as timely solve an issue.